Researchers from Ewha Womans University, Seoul, South Korea, have proposed a machine learning technique for detecting surface cracks on fire damaged concrete. Even though concrete is known to possess good fire resistance, concrete structures are damaged when exposed to fire. Some of the defects usually observed when concrete is exposed to fire are change in colour, deflection, and cracking/spalling. Therefore, if a structure must be reused or repaired after a fire event, it is important to assess or investigate the extent of damage that has been done.
On the rationale for the study, the authors said,
One of the common investigation methods (of fire damaged structures) is optical observation of crack and deformation from the fire damaged structures. It would be cost effective if such optical observation is done quantitatively without requiring expensive testing machines or man power. Moreover, it would be very powerful if the crack information can be used as a guide to evaluate the performance of fire damaged concrete structures.
The authors employed a deep learning technique called Convolutional Neural Network (CNN) to detect surface cracks in fire damaged reinforced concrete beams. The results and findings were published in International Journal of Concrete Structures and Materials (Springer) in the year 2020. CNN is a deep learning technique that is primarily used for analyzing intricate structures of high-dimensional data such as high defnition (HD) images and videos. This method has been applied by some other researchers on damage assessment of concrete structures.
To carry out the study, the authors modelled five reinforced concrete beams of dimensions 250mm x 450mm x 5000 mm, reinforced with 3D19 at the bottom and 2D19 at the top. Moreover, stirrups of D10@150 c/c spacing were used to prevent shear failure when subjected to loading. The beams were subjected to variable fire duration/exposure time under sustained load, and heated according to the time-temperature curve developed by the International Standard Organisation.
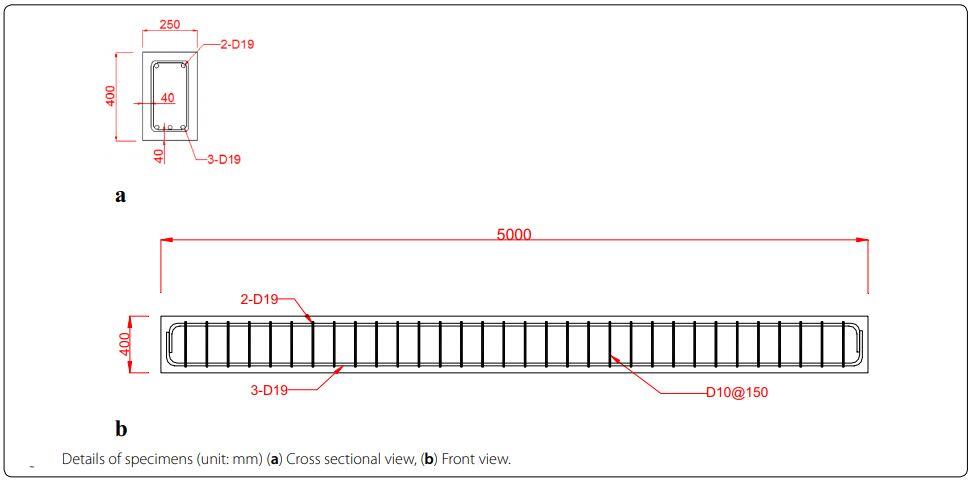
After the fire test, digital cameras were used to capture the surface of the concrete beams, and the images subjected to CNN architecture developed by the authors for training and testing. Subsequently, the study investigated the ratio of the number of pixels obtained from the CNN model to the crack length obtained from the optical observation, in order to see if consistency of the ratios can be found. It was observed that the ratios are almost same among the specimens having different variables. This tells that the proposed CNN method recognizes cracks of the fire damaged concrete beams from the surface images and follows similar changing tendencies of total crack lengths obtained from the optical observations.
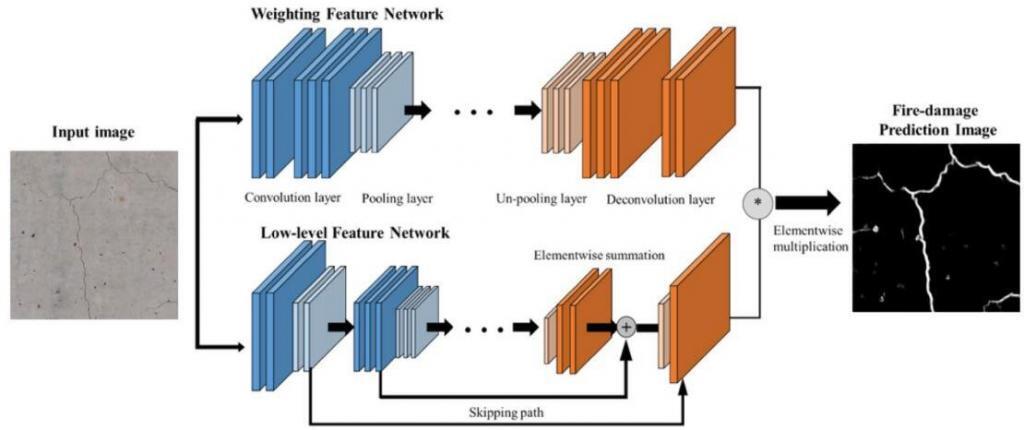
From their results, they concluded that the temperatures obtained from the thermocouples inside the beams are significantly related to total crack lengths of fire damaged beams rather than crack lengths at each zone. Also, they observed that there are strong relationships between the temperature and the number of pixels obtained from the proposed CNN model.
The limitation of the study is that the proposed CNN model is not able to capture crack depth and width. Nonetheless the number of pixels was found to be related to thermal-structural behaviors to some degree.
The findings of this article has been published on www.structville.com because it is licensed under a Creative Commons Attribution 4.0 International License, which permits use, sharing, adaptation, distribution and reproduction in any medium or format, as long as you give appropriate credit to the original author(s) and the source, provide a link to the Creative Commons licence, and indicate if changes were made. To view a copy of this licence, visit http://creativecommons.org/licenses/by/4.0/
Reference
[1] Ryu E., Kang J., Lee J., Shin Y., Kim H. (2020): Automated Detection of Surface Cracks and Numerical Correlation with Thermal-Structural Behaviors of Fire Damaged Concrete Beams. International Journal of Concrete Structures and Materials 14(12). https://doi.org/10.1186/s40069-019-0387-3